
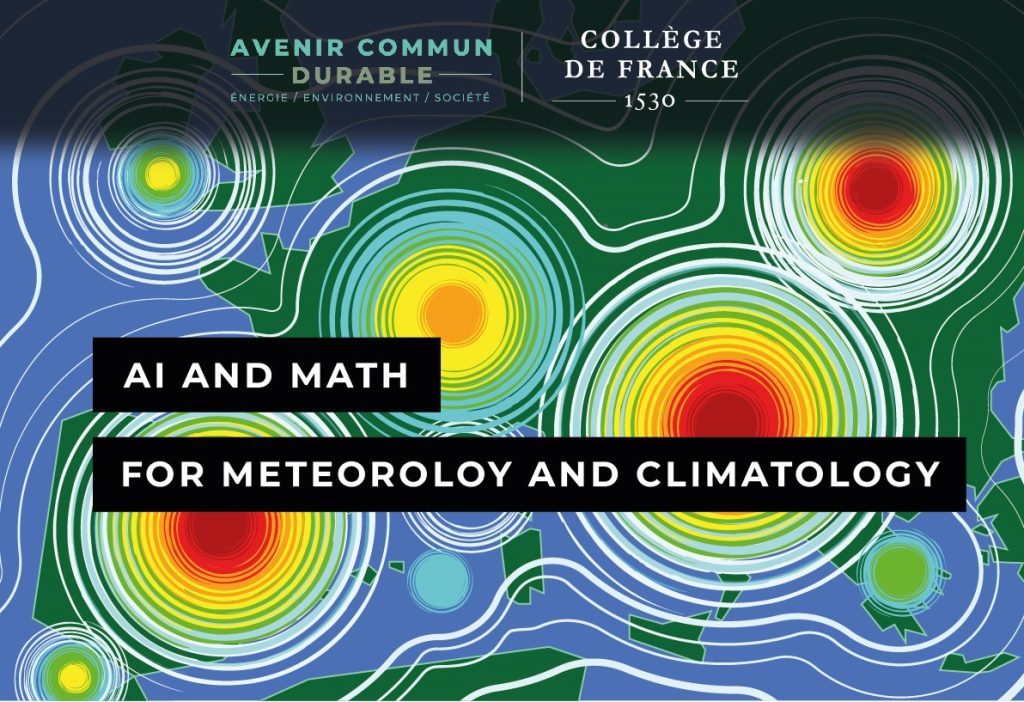
[Les interventions se feront en anglais.]
CONFERENCES
5 MAY / 9H00 > 17H30
Collège de France
11 place Marcelin Berthelot – 75005 Paris
Free to enter, depending on availability
Recent advances in artificial intelligence (AI) have produced unexpected and impressive results for weather forecasting, despite the complexity of these multi-scale phenomena. AI is also playing an increasingly important role in climatology. These results raise profound questions about modelling. On the one hand, we know the physics equations that have so far been used in large-scale numerical models. On the other hand, many physical parameters are unknown, for example at interfaces, which motivates a learning approach based on past data. We can also learn the evolution equations indirectly, eliminating the need for physical modelling. The approaches developed in AI in recent years oscillate between these two strategies.
This conference will present the state of the art at the interface between mathematics, physics and statistical learning using deep neural networks. It will highlight the advantages and disadvantages of the different modelling strategies, as well as the a priori incorporated in the form of equations or algorithmic architectures, in relation with physics. An objective is to encourage a dialogue between mathematicians, AI researchers, meteorologists and climatologists.
—
9H00 > 9h10: Stéphane Mallat, Collège de France
Introduction
9H10 > 10H10: Michael Brenner, Harvard University
The neural GCM, and other remarks
10H10 > 11H10: Thomas Dubos, École Polytechnique
Hamiltonian insights and the challenge of unresolved processes in geophysical models
11H30 > 12H30: Laure Zanna, New York University
Reshaping climate modelling with AI
14H00 > 15H00: Remi Lam, Massachusetts Institute of Technology
Learning global weather forecasting from data
15H00 > 16H00: Claire Monteleoni, INRIA Paris
Confronting climate change with generative and self-supervised machine learning
16H20 > 17h20: Marc Bocquet, École des Ponts
Artificial intelligence for geophysical data assimilation